목록논문 (14)
운동하는 공대생
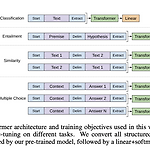
논문 https://www.cs.ubc.ca/~amuham01/LING530/papers/radford2018improving.pdf 1. Introduction 저자는 unsupervised 학습에 대한 어려움을 이야기를 했다. 그게 두 가지가 있는데 최적화를 어떻게 해야 하는지 문제와 데스크마다 변환하는 최적의 방식이 다르다는 문제가 있다. 그래서 이 논문에서는 semi-supervised 방식을 사용하여 이 문제를 해결했다고 말한다. 저자는 semi-supervised와 supervised fine-tuning을 결합하여 사용한다고 말했다. 두 가지의 단계로 훈련을 진행했는데 unlabeled 데이터에 대한 파라미터를 선행 학습하고 그 이후에는 이 파라미터를 가지고 추가적인 supervised 한 ..
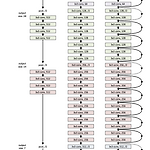
논문 https://arxiv.org/abs/1512.03385v1 Deep Residual Learning for Image Recognition Deeper neural networks are more difficult to train. We present a residual learning framework to ease the training of networks that are substantially deeper than those used previously. We explicitly reformulate the layers as learning residual functions with arxiv.org 0. Abstract 저자는 DNN 모델을 훈련을 할 때 residual functio..
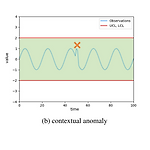
0. Intro 이 논문에서는 먼저 Time-series 데이터에서 Anomaly Detection을 하는 과제를 수행할 때 기본적인 데이터에 대한 특징이나 모델이 고려해야 하는 사항들에 대한 가이드라인을 제시를 해주었다. https://ieeexplore.ieee.org/document/9523565 Deep Learning for Anomaly Detection in Time-Series Data: Review, Analysis, and Guidelines As industries become automated and connectivity technologies advance, a wide range of systems continues to generate massive amounts of dat..
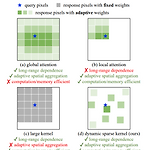
논문 - https://arxiv.org/abs/2211.05778 InternImage: Exploring Large-Scale Vision Foundation Models with Deformable Convolutions Compared to the great progress of large-scale vision transformers (ViTs) in recent years, large-scale models based on convolutional neural networks (CNNs) are still in an early state. This work presents a new large-scale CNN-based foundation model, termed arxiv.org 1. In..
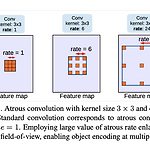
논문 - https://arxiv.org/abs/1706.05587v3 Rethinking Atrous Convolution for Semantic Image Segmentation In this work, we revisit atrous convolution, a powerful tool to explicitly adjust filter's field-of-view as well as control the resolution of feature responses computed by Deep Convolutional Neural Networks, in the application of semantic image segmentatio arxiv.org 1. Intro 기존의 컨볼루션 기반의 모델들은 지역..
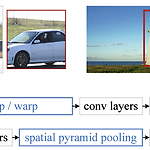
https://arxiv.org/abs/1406.4729v4 Spatial Pyramid Pooling in Deep Convolutional Networks for Visual Recognition Existing deep convolutional neural networks (CNNs) require a fixed-size (e.g., 224x224) input image. This requirement is "artificial" and may reduce the recognition accuracy for the images or sub-images of an arbitrary size/scale. In this work, we equip th arxiv.org 1 Intro 논문에서 제시한 문제..